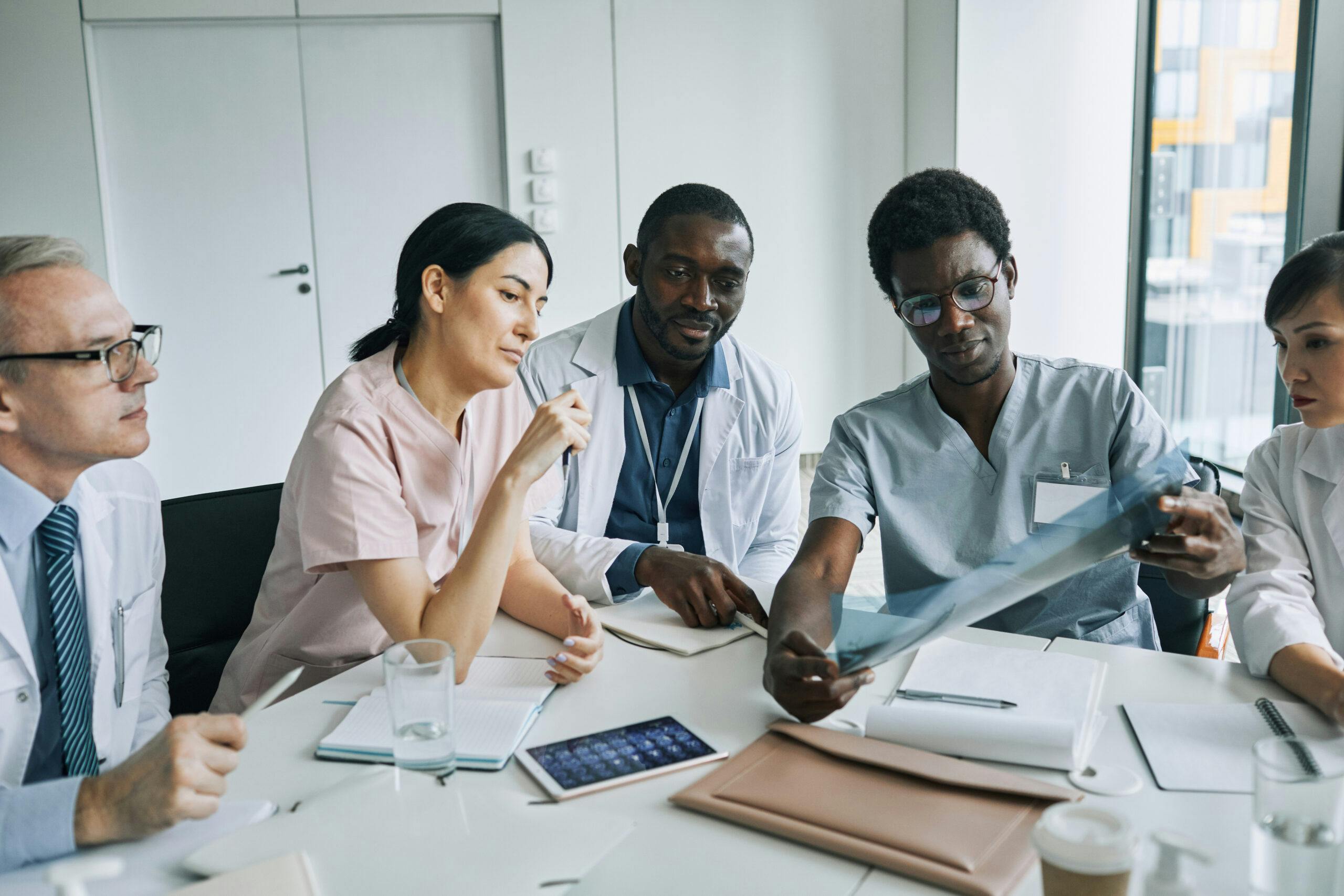
Featured Resource
Patient-Centric Digital Endpoints of Function for AD Research
FDA’s Patient-Focused Drug Development initiatives require that new evidence-generation tools are rooted in meaningful aspects of the patient experience.
Read MoreItems Per PageShowing 1 - 10 of 0